LAION and the Challenges of Preventing AI-Generated CSAM
Ritwik Gupta / Jan 2, 2024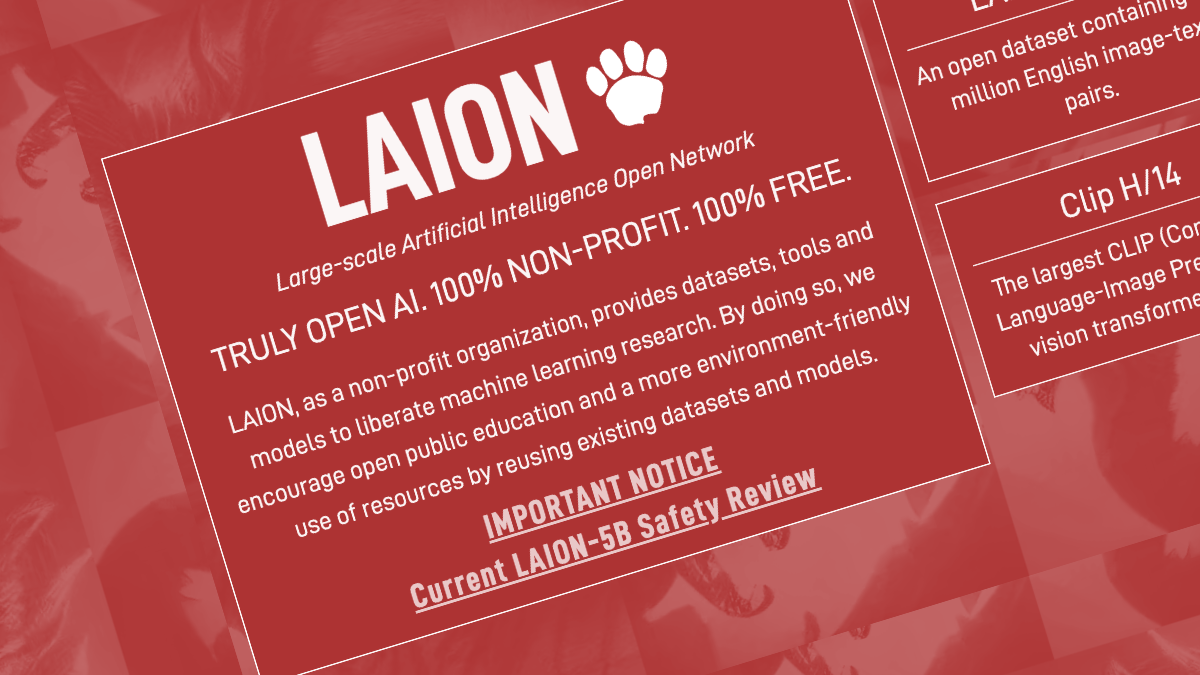
The current homepage of LAION, the nonprofit organization hosting LAION-5B, depicts a notice that the dataset is under a safety review.
Generative AI has been democratized. The toolkits to download, set up, use, and fine-tune a variety of models have been turned into one-click frameworks for anyone with a laptop to use. While this technology allows users to generate and experiment with a wide range of content, without guardrails it also allows for the generation of incredibly harmful material.
Last month, the Stanford Internet Observatory (SIO) released a report identifying Child Sexual Abuse Material (CSAM) in the Large-scale Artificial Intelligence Open Network’s (LAION) LAION-5B, a popular AI image training database. Building upon prior work, the report and ensuing press coverage highlighted an ugly and disturbing side of the generative AI boom and the challenges that face law enforcement, governments, and private industry in prosecuting, countering, and creating legislation around AI-Generated CSAM.
Law enforcement agencies and policymakers, already overwhelmed by the sheer volume of digital CSAM, are finding the rapid proliferation and the capabilities of generative AI daunting. These advanced systems can not only replicate existing CSAM but also generate new instances that do not involve direct human victims, complicating legal responses. The implications are profound: if a model is trained using data that includes original CSAM, its weights might replicate or even generate new CSAM, leading to a form of digital revictimization that is complex and pervasive. The LAION finding is particularly alarming—the dataset underpins widely used models such as Stable Diffusion 1.5 and Midjourney and was found to contain no less than 1,679 instances of CSAM, as identified by SIO.
Considering the technical nature of these models, particularly the way model weights might encapsulate actual CSAM, there is an urgent need to reassess how these are viewed under the law. Current legal frameworks need to evolve to address the nuances of both non-visual depictions of CSAM and synthetic CSAM, recognizing the potential of these models to harbor and perpetuate abuse. A concerted effort among governmental bodies, the private sector, and academia is imperative to guide the development of generative AI responsibly and ethically.
Background - Generative AI
Recent progress in generative AI has enabled the layperson to generate realistic artwork, images, and videos from text prompts. The latest generative AI models are called “diffusion models,” born from academic research at the University of California, Berkeley and Stanford University. In terms of useful background, a “model” is composed of a set of code that defines an “architecture” and a set of “weights” that define how a text prompt is turned into a meaningful image. Think of the “architecture” as the chassis of a car and the “weights” as the fuel: the chassis is useless without the fuel. These models are trained on datasets curated by scraping a massive amount of imagery and related text from the Internet—with different models from different firms like OpenAI or Stability AI using data collected through different sources. This enables these models to be generalist image generators: they generate common items well, while niche, specific items are often subpar.
To make these models work for more specific prompts, users can collect a small set of images and captions on a topic and “fine-tune” the model, therefore modifying the weights (but not the architecture) of the model to generate images tailored to their specifications.
Given a text prompt, any common smartphone on the market today can generate a realistic image in under 12 seconds. This time limit is decreasing rapidly as AI research progresses. Both the architectures and fine-tuned weights for many models are available for download to anyone around the world. Weights for specific topics such as “chibi anime”, “hyperrealistic portraits”, “lo-fi artist”, and more have been fine-tuned and made easily accessible.
Moreover, an unsettling aspect of these models is their capacity to “memorize” their training data, meaning that the weights of a trained model contain latent representations of the original data, which can then be reconstructed to produce the original or similar content. The issue becomes particularly alarming when considering the generation of CSAM. Since models can effectively memorize and potentially recreate the original training data, the weights of these models, when trained or fine-tuned on CSAM, are not just abstract numbers but may represent potential reproductions of real and harmful content. Recognizing the weights as potential reproductions of CSAM is crucial in understanding and legislating against the perpetuation of CSAM through these technologies.
Cases of Concern
To grasp the gravity and complexity of this issue, it is essential to understand the mechanisms by which malicious actors can harness generative AI to produce CSAM. Typically, this involves collecting CSAM, fine-tuning a generative AI model with this data, and then using the resulting model weights as a generative function to continually produce new instances of CSAM. Publicly accessible generative AI models, like Stable Diffusion XL1.5 and MidJourney, have been documented to have been trained on datasets containing CSAM such as LAION-5B, raising significant concerns.
Let's delve deeper into two illustrative cases:
Case 1: Fine-tuning a generative AI model to generate CSAM
Consider an individual aiming to generate CSAM. They begin by downloading a foundational generative AI model—typically designed to produce benign imagery—from the Internet. Armed with a collection of CSAM and using readily available open-source toolkits, this individual then fine-tunes the generative AI model, thereby creating a set of model weights that are optimized to produce CSAM. Consequently, the individual is in possession of several enabling items: the original CSAM, a model capable of generating both replicas of CSAM and novel synthetic CSAM, and the potential to generate a substantial volume of this illegal material with minimal effort.
Case 2: Downloading a generative AI model to generate CSAM
In this scenario, an individual acquires a model already fine-tuned for CSAM generation, possibly through downloading from the Internet or via transfer of files through physical media.
Through the use of widely available toolkits, this individual can generate a large volume of CSAM with ease. Unlike in the first case, the individual here does not possess physical CSAM images but rather the model weights derived from such material. These weights, however, are capable of containing near-perfect representations of the original CSAM, albeit in a transformed or scrambled form.
As mentioned above, generative AI models have the disconcerting ability to "memorize" their training data, meaning that when models are trained or further fine-tuned on CSAM, they inherently possess the ability to produce near exact replicas of the original abusive images within the weights. In this second case, while the individual does not hold the original CSAM physically, the internal representations within the model weights effectively constitute duplications of CSAM. This implies that each use of these weights to generate new CSAM indirectly contributes to the revictimization of individuals from the original CSAM, as their likenesses are exploited to create new, realistic instances of child sexual abuse.
Law Enforcement Challenges
In this changing environment, law enforcement is scrambling to keep up—both in the United States and around the globe. In the US, 18 U.S. Code § 2256 defines “child pornography” as “any visual depiction, including any photograph, film, video, picture, or computer or computer-generated image or picture, whether made or produced by electronic, mechanical, or other means, of sexually explicit conduct.” Furthermore, it defines the production of child pornography to be “producing, directing, manufacturing, issuing, publishing, or advertising” child pornography. Additionally, the PROTECT Act of 2003 outlaws any “drawing, cartoon, sculpture, or painting” that “depicts a minor [or someone appearing to be a minor] engaging in sexually explicit conduct.”
Laws written years ago must be updated. Under current interpretations of 18 U.S. Code § 2256, model weights likely do not represent a “visual depiction” of child pornography. Additionally, model weights do not appear to contain an “identifiable minor,” the definition under the PROTECT Act of 2003. Therefore, under current law, model weights derived from CSAM are not considered to be covered items under US statutes. Additionally, the above laws should be updated to place restrictions on automated tools that are primarily used to generate CSAM.
Moving forward, care must be taken to address loopholes in any proposed amendments. For example, a “second generation” model might be trained to reconstruct the outputs of a “first generation” model that was trained on original CSAM. As this model is being trained on outputs that are realistic, a “second generation” model could still be capable of generating realistic CSAM. However, the weights of a “second generation” model would not be derived from original CSAM—just synthetic, photo-realistic images. Ensuring that such loopholes do not exist will require a layered redefinition of synthetic CSAM and the tools used to generate it.
Responding to the Insatiable Need for Data at Any Cost
The LAION incident represents a serious misstep. The field of AI must do better to avoid a repetition of a failure of this scale in the future. Immediately, any person or organization involved with generative AI work should conduct a thorough inventory of their servers and identify if and where they possess affected datasets along with models that have been trained or otherwise derived from these models.
Additionally, the field of AI as a whole (including private industry, academia, and government) must create a standardized process for web-scale data curation that includes verifiable checks for harmful material and copyrighted material. Companies and academics are curating datasets larger than LAION-5B which almost certainly contain CSAM, necessitating the creation of a process that ensures that this does not occur again in the future.
Academia, in particular, must give more substantial consideration to the datasets that it accepts for usage in the field. LAION-5B was submitted to the highly influential Neural Information Processing Systems (NeurIPS) conference for peer review, where it was accepted and awarded the best paper in the “datasets and benchmarks” track. The reviews for the paper highlighted severe ethical concerns around copyrighted and harmful materials. Yet, the ethics reviewers for NeurIPS recommended that the paper be accepted after acknowledging that if NeurIPS did not accept the paper it would still be shared elsewhere and distributed. The bar for acceptance to AI’s most prestigious venues must be higher.
Academia and industry must also collaborate to establish methods by which trained models can be automatically vetted against databases such as PhotoDNA to ensure that their weights do not contain memorized CSAM, and to verify that models cannot create synthetic CSAM. Zero-knowledge proofs of model training (zkPoT) represent a promising start in this direction. Using a zkPoT, proofs for otherwise “black-box” models can be provided to auditors who can verify that the model was not trained on known instances of CSAM.
Players in the field of AI have been curating and releasing large datasets for years with no checks and balances in place. Indeed, many companies allow users to produce and distribute generative AI weights. As a consequence, there could be industry pushback against any legislation that attempts to limit the use of generative AI or adds a significant barrier to the commercialization of such software. By targeting the weights of the models themselves rather than the generated images output by the models, there is likely an easier path to enforcement under the First Amendment.
Lawmakers must act as well. US code must be amended to include non-visual depictions of CSAM as covered material. Additionally, the PROTECT Act should be updated to criminalize the creation, possession, and distribution of models that have been trained primarily for the creation of CSAM. To accomplish this in a manner that is free of loopholes, synthetic CSAM must also be re-defined in response to advances in technology. Strict liability for the release of unvetted web-scale data sources must also be established. This will require legislation and battles in court.
Finally, investigative procedures at law enforcement agencies, including the US Federal Bureau of Investigation and other agencies around the world, need comprehensive updates to address the nuances of generative AI in the furtherance of crimes including CSAM. This involves extending the scope of search warrants to rigorously scrutinize generative AI models and the associated weights when such materials are discovered on a suspect's computer. Beyond procedural updates, there's a pressing need to enhance the technical acumen of law enforcement personnel. Training programs specifically tailored to understanding and investigating the intricacies of generative AI technologies are crucial. This will empower law enforcement to effectively identify, trace, and prosecute the misuse of AI in generating and distributing CSAM, ensuring that officers are not only equipped with the necessary legal tools but also the technical expertise to tackle these complex challenges.
Together, these measures serve as a strong start to ensuring the field of AI is responsible for protecting against the curation, generation, and distribution of CSAM and other harmful material.
Authors
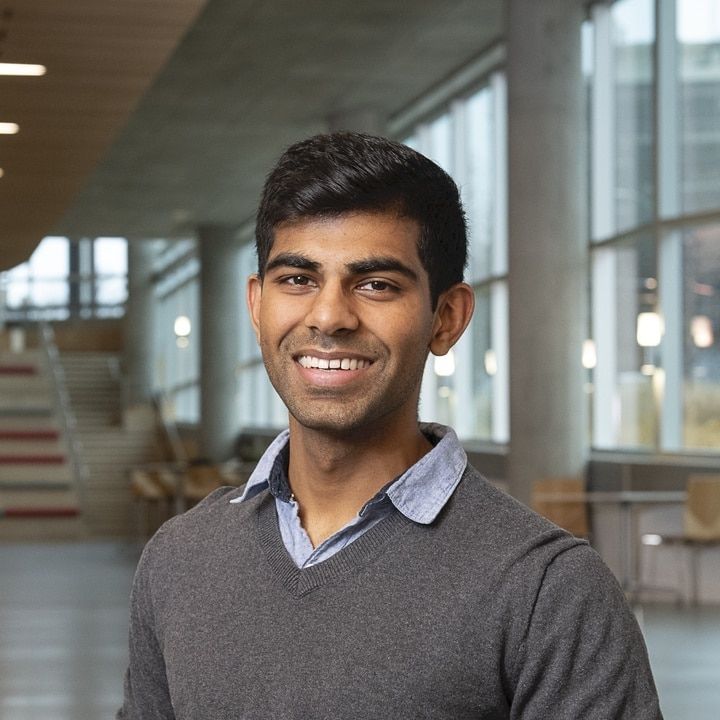